Exploring Generative AI Solutions for Your Business
Generative AI projects are inherently more complex, carrying a higher degree of uncertainty and increased risk. However, the potential rewards for successful projects are substantial, driving innovation across diverse industries. If you’re a visionary entrepreneur planning to launch a startup that could benefit from the capabilities of Generative AI, it’s crucial not to be discouraged by current limitations. Instead, focus on finding ways to make your ideas work. It’s important to note that the failure of Generative AI project ideas often results not from errors in data processing, algorithms, or model selection but rather from a lack of proper resources, expertise, or strategy from the project’s inception.
Initiating a Generative AI project with a well-thought-out approach is important for success. Seeking guidance from Generative AI experts early in the process can provide the confidence needed to move forward. On the following pages, we will explore how businesses can collaborate with experts to validate and implement Generative AI use cases through a systematic and scientific approach.
Why Validate Your Generative AI Ideas?
Across the globe, technology startups are harnessing the power of Generative AI and artificial intelligence to address previously unsolved problems. Being an early mover in the AI space, armed with high-quality training data for accurate predictions, can lead to success. However, determining the right problem to apply Generative AI to is the first critical step.
In software development, lean practices to reduce risks early in the development process are essential. The same principle applies to Generative AI projects, where the investment of both time and money is significant. It’s crucial to avoid finding oneself deeply engaged in a full-scale Generative AI project only to realize, upon completion, that the investment should have been directed differently.
Unraveling Unknowns
Unlike classical software engineering, where feasibility analysis is based on the current state of the art, Generative AI introduces unique challenges due to numerous unknowns. The success of a Generative AI solution depends on factors such as the quality of the data and the underlying model idea. There’s a possibility that the proposed solution may not work due to technical limitations in obtaining high-predictive-power data, the complexity of the problem exceeding the capabilities of neural networks, or the need for computational capabilities that currently need to be more attainable.
Team composition
A skilled team of experienced Data Scientists is fundamental to the success of your project. The careful selection of methodologies, coupled with an intuitive sense of feasibility, has the potential to save you weeks of unproductive efforts. This strategic approach not only accelerates the development process but also produces better results compared to the traditional build-verify loop. In the realm of AI projects, steering clear of the pitfalls of a cyclical and resource-draining process ensures that your budget is optimized for meaningful advancements rather than being consumed in a vicious loop of diminishing returns.
Idea validation
This is where business owners and startups often seek validation for their Generative AI ideas, looking for support and answers. Validation may require research or experimentation, necessitating the connection of the scientific and business worlds in Generative AI projects. To address this need, a roadmap has been developed, consisting of three concrete milestones:
- Generative AI Workshop
- Generative AI PoC (Proof of Concept)
- Generative AI MVP (Minimum Viable Product)
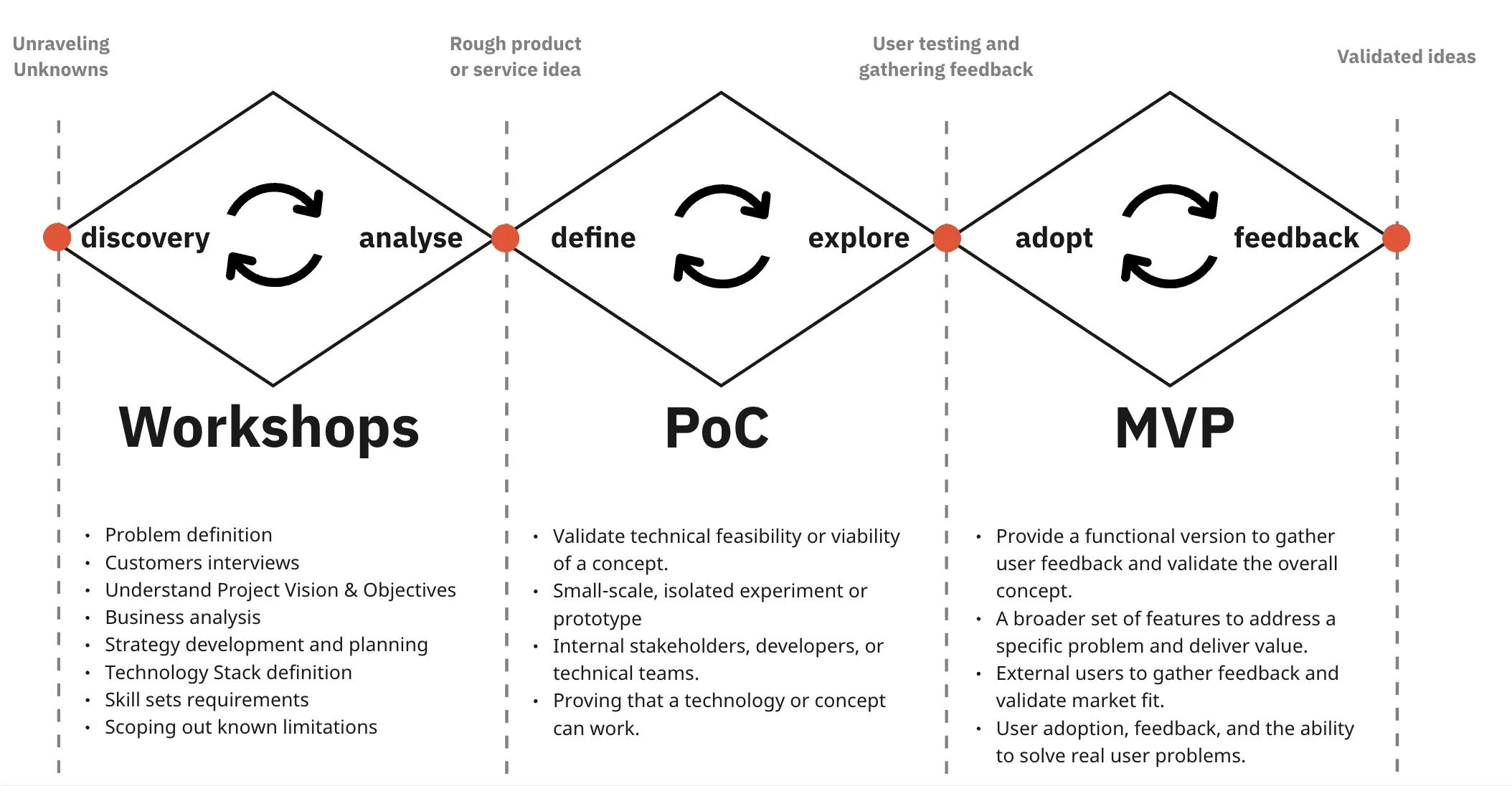
1) Generative AI Workshop
The first crucial step in the journey is to determine if your business idea holds promise in the context of Generative AI. While Generative AI can be a powerful solution, it may not always be the optimal one for a given problem. The workshop, spanning 1 to 2 days, is designed to create a space where business and scientific ideas converge to identify the most appropriate path forward.
Workshop Overview
- The workshop is intensive and resembles an interview, conducted by experienced machine learning engineers with specialized education in scientific fields.
- Understanding the client’s perspective is of utmost importance. The workshop involves asking the right questions about the idea’s origin, learning about the company and its market position, and aligning needs with the available science and machine learning technologies.
- Business analysis is integrated into the workshop, contributing a strategic perspective. It involves assessing the potential impact of AI/GenAI applications beyond what may be immediately apparent to the customer.
- After the initial day, a list of research problems is provided based on the discovered information. This list serves as a foundation for further exploration, addressing areas of concern, potential improvements, obstacles to overcome, or troubling questions in scientific theory or practice.
- The subsequent days focus on collaborative analysis, with machine learning engineers sharing insights and working together to find solutions. The goal is to achieve a clear understanding of the identified problems and develop better solutions.
Workshop Goal
- After 1-2 days of workshops, you will know whether Generative AI is a suitable solution or if there’s a better, more straightforward approach to achieving your goals.
- You will receive a Workshop Report within 5 working days, including your project description, a list of engineering competencies needed, an idea, plan, and time estimation for the Proof of Concept stage, and a draft of a Generative AI architecture for your project.
- Full transparency is maintained, allowing for adjustments to the approach based on the obtained results.
If the project is not well-suited for Generative AI, this will be communicated clearly.
2) Generative AI PoC (Proof of Concept)
If the Workshop indicates that your idea aligns well with Generative AI, the next step is to verify it through a Proof of Concept (PoC). The PoC involves building and training a model and demonstrating progress over one to six months. Regular meetings ensure continuous communication, allowing for swift adjustments when necessary.
PoC Overview
- Lasting up to six months, the Generative AI PoC involves regular meetings every two weeks to demonstrate progress.
- Data usage during the PoC stage is decided based on the outcomes of the Workshop. Existing example datasets may be recommended, or there might be a need to collect new data. The PoC stage includes assistance in data collection, providing proper gathering methodology, installing supporting software, and establishing a team of data analysts and engineers.
PoC Goals
- The primary objective is to determine if the proposed generative AI or machine learning concept is technically feasible and can deliver the desired outcomes.
- Focused on addressing core problems that require validation before progressing to the next stage
- Embrace change by systematically defining scenarios through a process of experimentation and iteratively refining initial ideas
- Validate the technical aspects of the project, ensuring that the chosen algorithms and models are suitable.
- Assess the availability and suitability of the required data for training and testing the generative AI model.
- Identify and assess potential risks and challenges associated with the implementation of the generative AI solution.
3) Generative AI MVP (Minimum Viable Product)
If the idea successfully progresses through the Workshop and PoC stages, it’s time to move forward and build the Generative AI software for the Minimum Viable Product (MVP). The MVP extends the validation process to the market by delivering a functional product for user testing and feedback.
MVP Overview
The goal of an MVP project is to efficiently and effectively introduce a product to the market, learn from user interactions, and iteratively enhance the product based on actual usage and feedback. It serves as a strategic approach to product development, balancing speed, resource efficiency, and user satisfaction.
MVP Process
Refined Problem Definition
- Use insights gained from the PoC to refine and clarify the problem definition.
- Clearly articulate the specific problem the MVP aims to solve based on PoC findings.
Data Validation and Model Enhancement:
- Validate the data used in the PoC and assess its suitability for the MVP.
- Enhance the machine learning model based on lessons learned during the PoC, addressing any identified limitations.
User Interface Optimization
- Optimize the user interface based on feedback and usability testing from the PoC.
- Ensure the interface aligns with user expectations and provides seamless interaction with the MVP features.
Scalability and Performance Planning
- Plan for scalability based on insights from the PoC, considering potential future growth.
- Address any performance considerations identified during the PoC to enhance the overall robustness of the MVP.
Summary
Testing your Generative AI idea at each stage of development pays off in the long run. Navigating the complex landscape of new algorithms requires informed tech choices. The collaborative path developed by working with clients establishes an environment where business and scientific ideas collide to define and implement Generative AI technology in the right applications.
If you’re interested in exploring Generative AI workshops, Proof of Concept, or MVP steps for your project, reach out by sending an email to sales@cobrick.com, and let’s apply science to your ideas!
Enjoyed this article? Don't miss out! Join our LinkedIn community and subscribe to our newsletter to stay updated on the latest business and innovation news. Be part of the conversation and stay ahead of the curve.
Transform your business with our expert
